TOP
Research
Research Teams
Next Generation High Performance Architecture Research Team
Next Generation High Performance Architecture Research Team
Japanese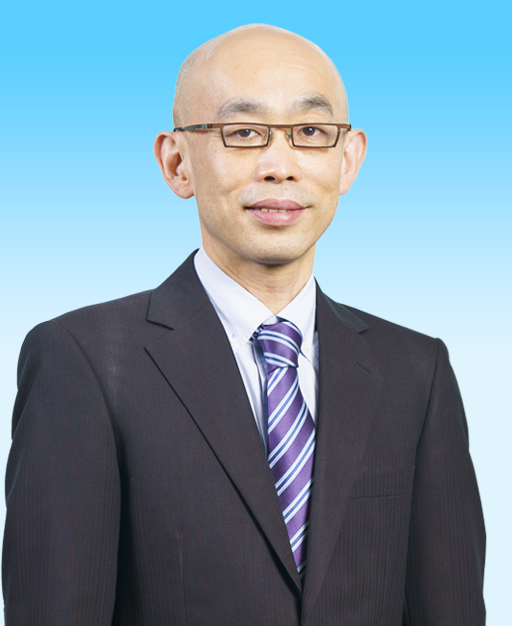
Team Principal Masaaki Kondo
masaaki.kondo[at]riken.jp (Lab location: Kobe)
- Please change [at] to @
- 2025
- Division Director, Next-Generation HPC Infrastructure Development Division, RIKEN R-CCS (-present)
- 2021
- Professor, Department of Information and Computer Science, Keio University (-present)
- 2021
- Professor, Department of Information and Computer Science, Keio University (-present)
- 2018
- Team Leader, Next Generation High Performance Architecture Research Team, RIKEN R-CCS (-present)
- 2014
- Visiting Researcher, Architecture Development Team of Flagship 2020 Project, RIKEN R-CCS
- 2013
- Associate Professor, Graduate School of Information Science and Technology, The University of Tokyo
- 2008
- Associate Professor, Graduate School of Information Systems, The University of Electro-Communications
- 2007
- Project Associate Professor, Research Center for Advanced Science and Technology, The University of Tokyo
- 2004
- Project Research Associate, Research Center for Advanced Science and Technology, The University of Tokyo
- 2003
- Research Associate, Core Research for Evolutional Science and Technology (CREST) Program, Japan Science and Technology Agency
- 2003
- Ph.D in Engineering, The University of Tokyo
Keyword
- Computer Architecture
- Supercomputer
- Parallel and distributed processing
- Non-von Neumann architecture
- Computing accelerator
Research summary
The continuous improvement in processing speed in high-performance computer systems such as the supercomputers K and Fugaku have been enabled by transistor scaling. This trend is known as Moore’s law. But Moore’s law is predicted to end in the near future. Hence, it is vital to research and develop novel and more efficient high performance computer systems if we are to continue realizing high performance computing in the future.
Based on the experience of hardware development and the existing software environment of the supercomputers K and Fugaku, we are researching and developing a next-generation high-performance computer architecture together with strategies to improve the power efficiency of exascale supercomputer systems. Currently, we are mainly focusing on non-von Neumann architectures such as systolic arrays and neuromorphic computers based on the latest advances in device technologies, architectures that can integrate next generation non-volatile memories and/or various types of accelerators into a general-purpose processor, the advancement of scientific simulations by accelerating machine learning computations, and hybrid computing architectures that combine the benefits of quantum computing and classical computing. And we are performing detailed co-design (coordinated design of hardware and software) evaluations of the computer architectures noted above as well as the co-design evaluations of algorithms that take advantage of them on the supercomputers K and Fugaku.
Main research results
Development of power management strategies for next-generation high performance computing systems
Power consumption is a prerequisite design constraint for developing exascale or next-generation computer systems. In order to maximize effective performance within a given power constraint, we need a new system-design concept in which the system’s peak power is allowed to exceed maximum power provisioning using adaptively controlling power knobs incorporated in hardware components so that effective power consumption is maintained below the power constraint. In such systems, it is indispensable to allocate the power budget adaptively among various hardware component such as processors, memories, and interconnects, or among co-scheduled jobs, instead of fully utilizing all available hardware resources.
To help resolve this challenge, we have devised a software framework for code optimization and system power management. For example, we have developed a variation-aware power budgeting scheme to maximize effective application performance, and in tests it produced a 5.4X speed increase compared to a variation-unaware power allocation scheme. Based on the Slurm workload manager, we have also developed a power-aware resource manager and a job scheduler to control power allocation among co-scheduled jobs. We have tested this framework on a large-scale HPC cluster system with about 1000 compute-nodes and showed that it can successfully manage the system’s power consumption below a given power constraint.
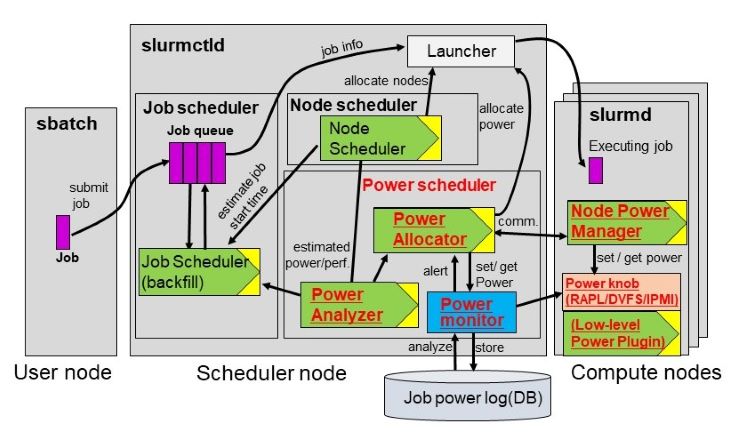
Representative papers
- Tsukada, M, Kondo, M., and Matsutani, H.:
OS-ELM-FPGA: An FPGA-Based Online Sequential Unsupervised Anomaly Detector"
The 16th International Workshop on Algorithms, Models and Tools for Parallel Computing on Heterogeneous Platforms (HeteroPar'18). (2018).
- Sakamoto, R., Patki, T., Cao, T., Kondo, M., Inoue, K., Ueda, M., Ellsworth, D., Rountree, B., and Martin Schulz.:
Analyzing Resource Trade-offs in Hardware Overprovisioned Supercomputers"
32nd IEEE International Parallel & Distributed Processing Symposium (IPDPS2018), 10 pages. (2018).
- Wada, Y., He, Y., Cao, T., and Kondo, M.:
"A Power Management Framework with Simple DSL for Automatic Power-Performance Optimization on Power-Constrained HPC Systems"
SupercomputingAsia 2018 (SCA18), 20 pages. (2018).
- Shresthamali, S., Kondo, M., and Nakamura, H.:
"Adaptive Power Management in Solar Energy Harvesting Sensor Node using Reinforcement Learning"
ACM Transactions on Embedded Computing Systems, Vol.16, No.5s, pp.181:1-181:21. (2017).
- Sakamoto, R., Takata, R., Ishii, J., Kondo, M., Nakamura, H., Ohkubo, T., Kojima, T., and Amano, H.:
"The Design and Implementation of Scalable Deep Neural Network Accelerator Cores"
IEEE 11th International Symposium on Embedded Multicore/Many-core Systems-on-Chip (MCSoC-17), 8 pages. (2017).
- Sakamoto, R., Cao, T., Kondo, M., Inoue, K., Ueda, M., Patki, T., Ellsworth, D., Rountree, B., and Schulz, M.:
"Production Hardware Overprovisioning: Real-world Performance Optimization using an Extensible Power-aware Resource Management Framework"
31st IEEE International Parallel & Distributed Processing Symposium (IPDPS2017). 10 pages. (2017).
- Cao, T., Huang, W., He, Y., and Kondo, M.:
"Cooling-Aware Job Scheduling and Node Allocation for Overprovisioned HPC Systems"
31st IEEE International Parallel & Distributed Processing Symposium (IPDPS2017), 10pages. (2017).
- Ohkubo, T., Tanaka, T., Sakamoto, T., Kondo, M., and Amano, H.:
"NAMACHA: A Software Development Environment for a Multi-Chip Convolutional Network Accelerator"
32nd International Conference on Computers and Their Applications (CATA'17). (2017).
- He, Y., Kondo, M., Nakada, T., Sasaki, H., Miwa, S., and Nakamura, H.:
"A Runtime Multi-Optimization Framework to Realize Energy Efficient Networks-on-Chip"
IEICE Transactions on Information and Systems, Vol.E99-D, No.12, pp.2881-2890. (2016).
- Inadomi, Y., Patki, T., Inoue, K., Aoyagi, M., Rountree, B., Schulz, M., Lowenthal, D., Wada, Y., Fukazawa, K., Ueda, M., Kondo, M., and Miyoshi, I.:
"Analyzing and Mitigating the Impact of Manufacturing Variability in Power-Constrained Supercomputing"
The International Conference for High Performance Computing, Networking, Storage and Analysis (SC15), 2 pages. (2015)