TOP
Research
HPC- and AI-driven Drug Development Platform Division
Past Units
Medicinal Chemistry Applied AI Unit (Honma)
Medicinal Chemistry Applied AI Unit (Honma)
Japanese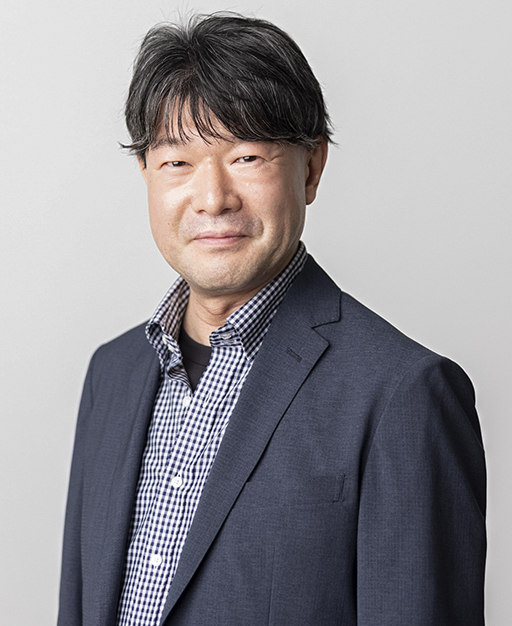
Unit Leader Teruki Honma
(Lab location: Yokohama)
- Please change [at] to @
- 2021
- Deputy division director, HPC and AI driven Drug Development Platform Division, RIKEN R-CCS/Unit leader, Medicinal Chemistry Applied AI Unit, RIKEN R-CCS (-present)
- 2018
- Deputy group director, RIKEN MIH/Unit leader,Medicinal Chemistry Data Intelligence Unit, RIKEN MIH
- 2010
- Manager, RIEKN DMP/Unit leader, Drug Discovery Computational Chemistry Platform Unit, RIEKN BDR (-present)
- 2008
- Team leader, Laboratory for Structure-Based Molecular Deaign RIKEN GSC (RIEKN BDR from 2018) (-present)
- 2007
- Senior scientist, RIKEN GSC
- 2004
- Senior principle scientist, Pfizer Japan Inc.
- 2001
- Obtained Ph.D.(Science) Department of Chemistry, Faculty of Science, Hokkaido University
- 1993
- Banyu Pharmaceutical Co.Ltd.
- 1993
- Obtained M.S. Department of Chemistry, Faculty of Science, Hokkaido University
Keyword
- In silico screening
- ADMET
- FMO method
- Artificial intelligence
- Deep learning
Research summary
At each stage of drug discovery, "hit to lead"(from initial hits to lead compounds showing in vivo efficacy) stage has become a bottleneck along with phase II clinical trials, and the stagnation of small molecule drug discovery in recent years. This unit aims to improve the time required and cost of the hit-to-lead and lead optimization process (so-called medicinal chemistry process) by AI. The cause of the increased time and cost of the medicinal chemistry process is the difficulty of optimizing potency and ADMET (pharmacokinetics and toxicity) at the same time. In collaboration with Kyoto University and LINC, we are building AI models for new structure generation, synthetic pathway prediction, and potency / ADMET prediction in order to speed up the medicinal chemistry process.
Main research results
- Accelerate new drug purchases by sharing data from several companies in production Life on arrival of completed client. RIKEN press release (Mar. 8, 2021)
- New dataset for drug design uses quantum mechanics-based interactions of new coronavirus proteins and drug candidates
. RIEKN press release (Apr. 18, 2020, Figure 1)
- Commercialization of drug discovery support informatics system construction business results. RIKEN press release (Mar. 18, 2019)
- FMO database(https://drugdesign.riken.jp/FMODB/)
. AMED BINDS news (Mar. 1, 2019)
- Honma T. ADMET prediction and its application to new structure proposal AI. MEDCHEM NEWS, 28(4), 167-174, 2018.
- Honma T. Aiming at structure proposal AI that enables simultaneous optimization of activity and efficacy and ADMET. Medicine and Drug Journal (Iyaku Journal), 54(9), 82, 2018.
- AMED cardiovascular databse (https://drugdesign.riken.jp/hERGdb/)
. AMED (Aug. 31, 2018)
Representative papers
- Watanabe C, Okiyama Y, Tanaka S, Fukuzawa K, Honma T.:
“Molecular Recognition of SARS-CoV-2 Spike Glycoprotein: Quantum Chemical Hot Spot and Epitope Analyses”
DOI: 10.1039/D0SC06528E (Edge Article) Chem. Sci., (2021). - Takaya D, Watanabe C, Nagase S, Kamisaka K, Okiyama Y, Moriwaki H, Yuki H, Sato T, Kurita N, Yagi Y, Takagi T, Kawashita N, Takaba K, Ozawa T, Takimoto-Kamimura M, Tanaka S, Fukuzawa K, Honma T.:
“FMODB: The World's First Database of Quantum Mechanical Calculations for Biomacromolecules Based on the Fragment Molecular Orbital Method”
J Chem Inf Model. 22;61(2):777-794. (2021). - Komura H, Watanabe R, Kawashima H, Ohashi R, Kuroda M, Sato T, Honma T, Mizuguchi K.:
“A public-private partnership to enrich the development of in silico predictive models for pharmacokinetic and cardiotoxic properties”
Drug Discov Today. 28:S1359-6446(21)00055-6. (2021) - Kato K, Honma T, Fukuzawa K.:
“Intermolecular interaction among Remdesivir, RNA and RNA-dependent RNA polymerase of SARS-CoV-2 analyzed by fragment molecular orbital calculation”
J Mol Graph Model. 100:107695. (2020). - Kato K, Masuda T, Watanabe C, Miyagawa N, Mizouchi H, Nagase S, Kamisaka K, Oshima K, Ono S, Ueda H, Tokuhisa A, Kanada R, Ohta M, Ikeguchi M, Okuno Y, Fukuzawa K, Honma T.:
“High-precision Atomic Charge Prediction for Protein Systems Using Fragment Molecular Orbital Calculation and Machine Learning”
J Chem Inf Model. 27;60(7):3361-3368. (2020) - Kojima R, Ishida S, Ohta M, Iwata H, Honma T, Okuno Y.:
“kGCN: a graph-based deep learning framework for chemical structures”
Journal of Cheminformatics 12: 1-10. (2020) - Ogura K, Sato T, Yuki H, Honma T.:
“Support Vector Machine model for hERG inhibitory activities based on the integrated hERG database using descriptor selection by NSGA-II”
Scientific reports, 9(1), 1-12. (2019). - Watanabe C, Watanabe H, Okiyama Y, Takaya D, Fukuzawa K, Tanaka S, Honma T.:
“Development of an automated fragment molecular orbital (FMO) calculation protocol toward construction of quantum mechanical calculation database for large biomolecules”
Chem-Bio Informatics Journal, 19, 5-18. (2019). - Yoshikawa N, Terayama K, Honma T, Oono K, Tsuda K.:
“Population-based de novo molecule generation, using grammatical evolution”
Chem Lett, 47(11), 1431-143. (2018). - Sato T, Yuki H, Ogura K, Honma T.:
“Construction of an integrated database for hERG blocking small molecules”
PLoS ONE, 13(7), e0199348. (2018).