TOP
Research
Research Teams
Data Assimilation Research Team
Data Assimilation Research Team
Japanese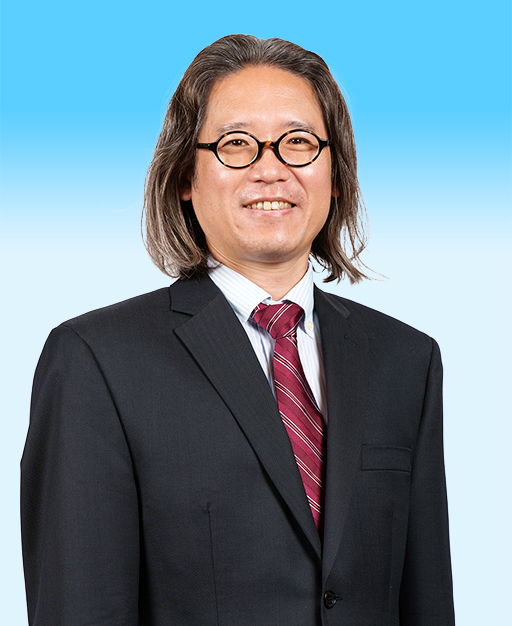
Team Leader Takemasa Miyoshi
takemasa.miyoshi[at]riken.jp (Lab location: Kobe)
- Please change [at] to @
- 2022
- Visiting Professor, Graduate School of Science, Kyoto University, Japan(-present)
- 2021
- Program Director, Japan Science and Technology Agency (JST)(-present)
- 2018
- Deputy Director, RIKEN interdisciplinary Theoretical and Mathematical Sciences (iTHEMS) Program, Japan(-present)
- 2018
- Chief Scientist, RIKEN Cluster for Pioneering Research, Japan(-present)
- 2018
- Affiliate Professor, Graduate School of Science, Kyoto University, Japan
- 2017
- Visiting Principal Scientist, Application Laboratory, JAMSTEC, Japan (-present)
- 2015
- Research Counselor, Servicio Meteorológico Nacional (National Meteorological Service), Argentina(-present)
- 2014
- Visiting Senior Scientist, Application Laboratory, JAMSTEC, Japan
- 2013
- Visiting Senior Research Scientist, Earth Simulator Center, JAMSTEC, Japan
- 2013
- Visiting Professor, University of Maryland, College Park, Maryland, USA(-present)
- 2012
- Team Leader, Data Assimilation Research Team, AICS (renamed R-CCS in 2018), RIKEN (-present)
- 2011
- Assistant Professor, University of Maryland, College Park, Maryland, USA
- 2009
- Research Assistant Professor, University of Maryland, College Park, Maryland, USA
- 2009
- Visiting Assistant Researcher, University of California, Los Angeles, California, USA
- 2005
- Scientific Official, Numerical Prediction Division, Japan Meteorological Agency, Japan
- 2005
- Ph.D. in Meteorology, University of Maryland, College Park, Maryland, USA
- 2004
- M.S. in Meteorology, University of Maryland, College Park, Maryland, USA
- 2003
- Graduate Student, University of Maryland, College Park, Maryland
- 2002
- Scientific Official, Numerical Prediction Division, Japan Meteorological Agency
- 2000
- Technical Official, Planning Division, Japan Meteorological Agency
- 2000
- B.S. in Physics, Faculty of Science, Kyoto University, Kyoto, Japan
Keyword
- Data Assimilation
- Numerical Weather Prediction
- Large Scale Simulation
- Ensemble Data Assimilation
- Natural disaster prediction
Research summary
Data assimilation (DA) is a cross-disciplinary science to synergize computer simulations and real-world data, using statistical methods and applied mathematics. As computers become more powerful and enable more precise simulations, it will become more important to compare the simulations with actual observations.
DA Team performs cutting-edge research and development on advanced DA methods and their wide applications, aiming to integrate computer simulations and real-world data in the wisest way. Particularly, DA Team tackles challenging problems of developing efficient and accurate DA systems for “big simulations” with real-world “big data” from various sources including advanced sensors. The specific foci include 1) theoretical and algorithmic developments for efficient and accurate DA, 2) DA methods and applications by taking advantage of the world-leading supercomputer and “big data” from new advanced sensors, and 3) exploratory new applications of DA in wider simulation fields. These advanced DA studies will enhance simulation capabilities and lead to a better use of high-performance computers.
Main research results
K computer and advanced weather radar come together to predict sudden torrential rains
Using the K computer, we carried out 100 parallel simulations of a convective weather system, using the nonhydrostatic mesoscale model with 100-meter grid spacing and assimilated data from a next-generation phased-array weather radar, which was implemented in the summer of 2012 by the National Institute of Information and Communications Technology (NICT) and Osaka University. This enabled us to produce a high-resolution three-dimensional distribution map of rain every 30 seconds—120 times more rapidly than the typical hourly updated systems operated at weather prediction centers around the world today.
To test the accuracy of the system, we attempted to model a real case—a sudden storm that took place on September 11, 2014, in Kobe. The simulations were tested without observational data input, as well as with observational data incorporated every 30 seconds on 100-meter and 1-kilometer grid scales. The simulations alone were unable to replicate the rain, while the simulation incorporating observational data enabled the computer to represent the actual storm. In particular, the simulation carried out with 100-meter grids produced an accurate replication of the storm when compared to actual observations.
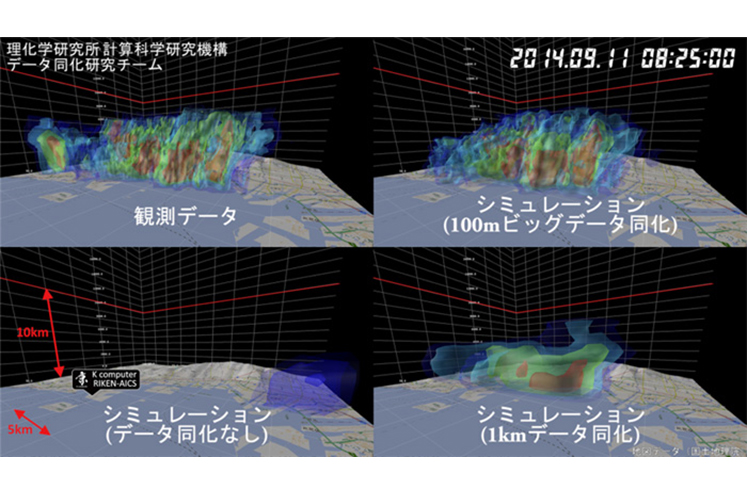
Representative papers
- Sun, Q., T. Miyoshi, S. Richard, 2023: Analysis of COVID-19 in Japan with extended SEIR model and ensemble Kalman filter., Journal of Computational and Applied Mathematics. doi.org/10.1016/j.cam.2022.114772
- Miyoshi, T. and Sun, Q., 2022: Control Simulation Experiment with the Lorenz's Butterfly Attractor, Nonlin. Processes Geophys., 29, 133-139, 2022. doi.org/10.5194/npg-29-133-2022
- Honda, T., A. Amemiya, S. Otsuka, G.-Y. Lien, J. Taylor, Y. Maejima, S. Nishizawa, T. Yamaura, K. Sueki, H. Tomita, S. Satoh, Y. Ishikawa, and T. Miyoshi, 2022: Development of the Real-Time 30-s-Update Big Data Assimilation System for Convective Rainfall Prediction with a Phased Array Weather Radar: Description and Preliminary Evaluation, J. Adv. Modeling Earth Systems, 14(6), e2021MS002823. doi:10.1029/2021MS002823
- Otsuka, S., S. Kotsuki, M. Ohhigashi, and T. Miyoshi, 2019: GSMaP RIKEN Nowcast: Global precipitation nowcasting with data assimilation. J. Meteor. Soc. Japan, 97, 1099-1117. doi:10.2151/jmsj.2019-061
- Honda, T., S. Kotsuki, G.-Y. Lien, Y. Maejima, K. Okamoto and T. Miyoshi, 2018: Assimilation of Himawari-8 All-Sky Radiances Every 10 Minutes: Impact on Precipitation and Flood Risk Prediction. J. Geophys. Res., 123, 965-976. doi:10.1002/2017JD027096
- Arakida, H., T. Miyoshi, T. Ise, S. Shima and S. Kotsuki, 2017: Non-Gaussian data assimilation of satellite-based Leaf Area Index observations with an individual-based dynamic global vegetation model. Nonlinear Processes in Geophys., 24, 553-567. doi:10.5194/npg-24-553-2017
- Miyoshi, T., G.-Y. Lien, S. Satoh, T. Ushio, K. Bessho, H. Tomita, S. Nishizawa, R. Yoshida, S. A. Adachi, J. Liao, B. Gerofi, Y. Ishikawa, M. Kunii, J. Ruiz, Y. Maejima, S. Otsuka, M. Otsuka, K. Okamoto, and H. Seko, 2016: "Big Data Assimilation" toward Post-peta-scale Severe Weather Prediction: An Overview and Progress. Proc. of the IEEE, 104, 2155-2179. doi:10.1109/JPROC.2016.2602560
- Miyoshi, T., M. Kunii, J. Ruiz, G.-Y. Lien, S. Satoh, T. Ushio, K. Bessho, H. Seko, H. Tomita, and Y. Ishikawa, 2016: "Big Data Assimilation" Revolutionizing Severe Weather Prediction. Bull. Amer. Meteor. Soc., 97, 1347-1354. doi:10.1175/BAMS-D-15-00144.1
- Miyoshi, T., K. Kondo, and K. Terasaki, 2015: Big Ensemble Data Assimilation in Numerical Weather Prediction. Computer, 48, 15-21. doi:10.1109/MC.2015.332
- Miyoshi, T., K. Kondo, and T. Imamura, 2014: The 10240-member ensemble Kalman filtering with an intermediate AGCM. Geophys. Res. Lett., 41, 5264-5271. doi:10.1002/2014GL060863
Annual Reports
Press Releases & Other News
- Release of JAXA-RIKEN Ocean Analysis through JAXA’s P-Tree System (Apr 24, 2023)
- RIKEN and JAXA collaborate to offer real-time rainfall forecasts
(Aug 20, 2020, RIKEN Website)
Members
Related Websites
Predicting sudden downpours(YouTube, approx. 2 minutes)