TOP
Research
HPC- and AI-driven Drug Development Platform Division
Medicinal Chemistry Applied AI Unit
Medicinal Chemistry Applied AI Unit
Japanese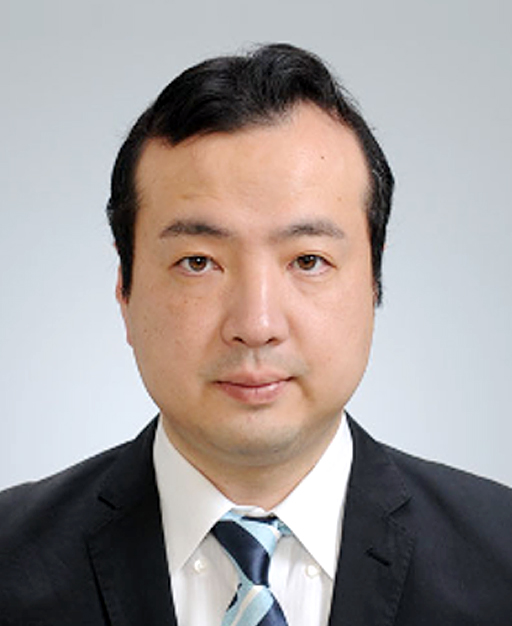
Unit Leader Kazuyoshi Ikeda
kazuyoshi.ikeda[at]riken.jp (Lab location: Yokohama)
- Please change [at] to @
- 2005
- Ph.D. in Life Science at Graduated School of Tokyo University of Pharmacy & Life Science
- 2005
- Computational Biology Research Center (CBRC), National Institute of Advanced Industrial Science and Technology (AIST) Postdoctoral Researcher
- 2005
- PharmaDesign Inc. Principal Scientist
- 2009
- EMBL-EBI Scientific Application Developer
- 2012
- Level Five Co.,Ltd.Principal Scientist & Team Leader
- 2016
- Keio University Faculty of Pharmacy Project Associate Professor
- 2020
- RIKEN Center for Computational Science(R-CCS), Senior Scientist
- 2024
- RIKEN Center for Computational Science(R-CCS), HPC- and AI-driven Drug Development Platform Division, Medicinal Chemistry Applied AI Unit, UnitLeader
Keyword
- In silico screening
- Database
- Artificial intelligence
- Deep learning
- Middle molecule
Research summary
At each stage of drug discovery, "hit to lead"(from initial hits to lead compounds showing in vivo efficacy) stage has become a bottleneck along with phase II clinical trials, and the stagnation of small molecule drug discovery in recent years. This unit aims to improve the time required and cost of the hit-to-lead and lead optimization process (so-called medicinal chemistry process) by AI. The cause of the increased time and cost of the medicinal chemistry process is the difficulty of optimizing potency and ADMET (pharmacokinetics and toxicity) at the same time. In collaboration with Kyoto University and LINC, we are building AI models for new structure generation, synthetic pathway prediction, and potency / ADMET prediction in order to speed up the medicinal chemistry process.
Representative papers
- Shimizu Y., Ohta M., Ishida S., Terayama K., Osawa M., Honma T., Ikeda K.
"AI-driven molecular generation of not-patented pharmaceutical compounds using world open patent data."
J Cheminform. 2023, 15 (1), 120. - Ikeda K., Maezawa Y., Yonezawa T., Shimizu Y., Tashiro T., Kanai S., Sugaya N., Masuda Y., Inoue N., Niimi T., Masuya K., Mizuguchi K., Furuya T., Osawa M.
"DLiP-PPI library: An integrated chemical database of small-to-medium-sized molecules targeting protein–protein interactions."
Frontiers in Chemistry 2023 10, 1090643. - Ikeda K., Kezuka Y., Nonaka T., Yonezawa T., Osawa M., Katoh E.
"Comprehensive Approach of 19F Nuclear Magnetic Resonance, Enzymatic, and In Silico Methods for Site-Specific Hit Selection and Validation of Fragment Molecules that Inhibit Methionine γ-Lyase Activity."
J Med Chem. 2021, 64(19):14299-14310.