TOP
Research
AI for Science Platform Division
Life and Medical Science Application Interface Platform Development Unit
Life and Medical Science Application Interface Platform Development Unit
Japanese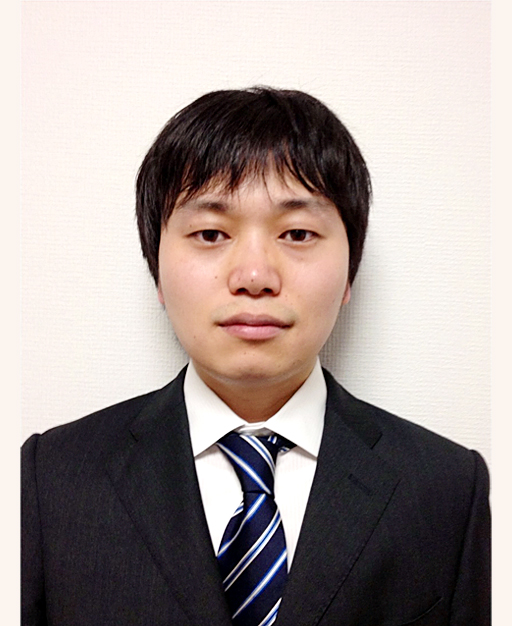
Unit Leader Yasuhiro MATSUNAGA
ymatsunaga[at]riken.jp (Lab location: Kobe)
- Please change [at] to @
- 2025
- Unit Leader, Life and Medical Science Application Interface Platform Development Unit, AI for Science Platform Division, R-CCS, RIKEN (-present)
- 2019
- Associate Professor, Graduate School of Science and Engineering, Saitama University (-present)
- 2018
- Research Scientist, Computational Biophysics Research Team, R-CCS, RIKEN
- 2016
- Researcher, Research Fellow of the Japan Science and Technology Agency (JST PRESTO)
- 2014
- Research Scientist, Computational Biophysics Research Team, AICS (renamed R-CCS in 2018), RIKEN
- 2011
- Special Postdoctoral Researcher, Computational Biophysics Research Team, AICS (renamed R-CCS in 2018), RIKEN
- 2008
- Postdoctoral Researcher, ISLiM Next-generation Integrated Simulation of Living Matter, RIKEN
- 2007
- Postdoctoral Researcher, Computational Life Science Laboratory, Yokohama City University
- 2007
- Department of Information and Media Science, Graduate School of Science and Technology, Kobe University
Keyword
- Integrated Modeling
- Data Assimilation
- Molecular Dynamics Simulation
Research summary
The structure and dynamics of biomacromolecules are not only crucial for maintaining life activities but are also related to diseases, making their detailed observation important. We are developing AI-based technologies to combine image data obtained from advanced experimental methods such as High-Speed AFM, Cryo-EM/ET, and state-of-the-art microscopy techniques with the three-dimensional structures of biomacromolecules. Additionally, we are developing application interfaces and workflow technologies to integrate measurement data with molecular structures and dynamics. Furthermore, we are developing an application interface platform to connect software developed for scientific research using high-performance computing with AI.
Representative papers
-
J. Jung, K. Yagi, C. Tan, H. Oshima, T. Mori, I.Yu, Y. Matsunaga,C. Kobayashi, S. Ito, D. Ugarte La Torre, Y. Sugita*
"GENESIS 2.1: High-Performance Molecular Dynamics Software for Enhanced Sampling and Free-Energy Calculations for Atomistic, Coarse-Grained, and QM/MM models",
J. Phys. Chem. B. 128, 6028-6048 (2024). -
T. Ishizone*, Y. Matsunaga, S. Fuchigami, and K. Nakamura
"Representation of Protein Dynamics Disentangled by Time-Structure-Based Prior",
J. Chem. Theory Comput. 20, 436-450 (2023). -
Y. Matsunaga*, S. Fuchigami, T. Ogane, and S. Takada
"End-to-End Differentiable Blind Tip Reconstruction for Noisy Atomic Force Microscopy Images",
Sci. Rep. 13, 129 (2023) -
Y. Matsunaga, M. Kamiya, H. Oshima, J. Jung, S. Ito, and Y. Sugia*
“Use of multistate Bennett acceptance ratio method for free-energy calculations from enhanced sampling and free-energy perturbation”,
Biophys. Rev. 14, 1503-1512 (2022). -
T. Ogane, D. Noshiro, T. Ando, A. Yamashita, Y. Sugita, and Y. Matsunaga*
“Development of hidden Markov modeling method for molecular orientations and structure estimation from high-speed atomic force microscopy time-series images”,
PLoS Comput. Biol. 18, e1010384 (23 pages) (2022). -
Y. Matsunaga, and Y. Sugita*
“Use of single-molecule time-series data for refining conformational dynamics in molecular simulations”,
Curr. Opin. Struct. Biol. 61, 153-159 (2020). -
Y. Matsunaga, and Y. Sugita*,
“Linking time-series of single-molecule experiments with molecular dynamics simulations by machine learning”,
eLife 7, e32668 (2018). -
Y. Matsunaga, and Y. Sugita*,
“Refining Markov State Models for conformational dynamics using ensemble-averaged data and time-series trajectories”,
J. Chem. Phys. 148, 241731 (2018).