TOP
Research
AI for Science Platform Division
AI for Science Foundation Model Research Team
AI for Science Foundation Model Research Team
Japanese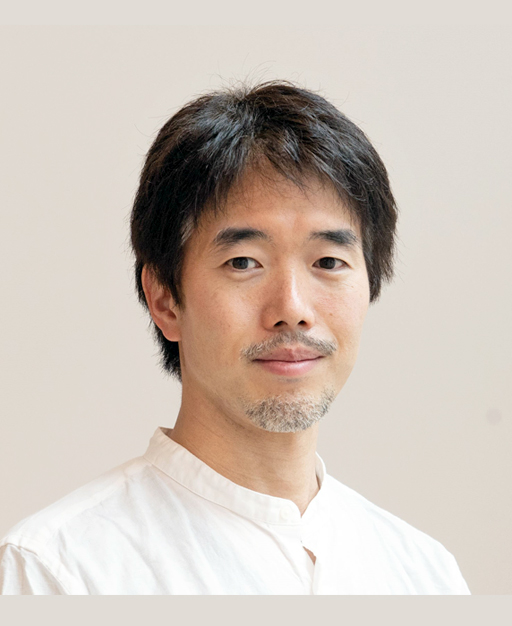
Team Principal Rio Yokota
rio.yokota [at] riken.jp (Lab location: Tokyo)
- Please change [at] to @
- 2025
- Team Principal, AI for Science Foundation Model Research Team, AI for Science Platform Division, RIKEN R-CCS (-present)
- 2024
- PresentProfessor, Institute of Integrated Research, Supercomputing Research Center, Institute of Science Tokyo
- 2023
- Professor, Global Scientific Information and Computing Center, Tokyo Institute of Technology
- 2015
- Associate Professor, Global Scientific Information and Computing Center, Tokyo Institute of Technology
- 2011
- Research Scientist, Applied Mathematics and Computer Science, King Abdullah University of Science and Technology
- 2010
- Post-doctoral Research Associate, Department of Mechanical Engineering, Boston University
- 2009
- Post-doctoral Research Associate, Department of Mathematics, University of Bristol
- 2009
- School of Science for Open and Environmental Systems (PhD), Graduate School of Science and Technology, Keio University
Keyword
- Ai for Science
- Foundation Model
- Reasoning Model
- Scalable Deep Learning
- Agentic AI
Research summary
The Advanced General Intelligence for Science Program (TRIP-AGIS) aims to contribute to the dramatic acceleration of the scientific research cycle and the broadening of the scope of exploration within scientific fields, by developing and applying advanced technologies based on artificial superintelligence. The AI for Science Foundation Model Research team aims to develop technology for efficiently training and deploying foundation models with advanced reasoning capabilities, which can assist scientists with tasks such as, proposal of research concepts, planning of experimental programs, development of research code, conducting experiments and simulations, and the analysis of experimental results. To achieve this, we will 1) seek appropriate interfaces and interactions for the foundation models in each scientific field, 2) improve the architecture of the foundation models based on this, 3) develop evaluation benchmarks for scientific domains, and 4) maintain the software infrastructure to enable training and inference to be carried out at maximum speed on next-generation supercomputers.
Representative papers
-
Nakamura, T., Mishra, M., Tedeschi, S., Chai, Y., Stillerman, J. T., Friedrich, F., Yadav, P., Laud, T., Chien, V. M., Zhuo, T. Y. et al.
Aurora-M: Open Source Continual Pre-training for Multilingual Language and Code
The 31st International Conference on Computational Linguistics (COLING), Industry Track, Abu Dhabi, UAE, Jan. 2025. -
Niwa, K., Ishii, H., Sawada, H., Fujino, A., Harada, N., Yokota, R.
Natural Gradient Primal-Dual Method for Decentralized Learning
Transactions on Signal and Information Processing over Networks, 10, 417-433, https://doi.org/10.1109/TSIPN.2024.3388948 (2024). -
Ma, Q, Yokota, R.
An Inherently Parallel H^2-ULV Factorization for Solving Dense Linear Systems on GPUs
International Journal of High Performance Computing Applications, 38(4), https://doi.org/10.1177/1094342024124202 (2024). -
Ootomo, H., Ozaki, K., Yokota, R.
DGEMM on Integer Matrix Multiplication Unit
International Journal of High Performance Computing Applications, 38(4), https://doi.org/10.1177/10943420241239588 (2024). -
Okazaki, N., Hattori, K., Hirai, S., Iida, H., Ohi, M., Fujii, K., Nakamura, T., Loem, M., Yokota, R., Mizuki, S.
Building a Large Japanese Web Corpus for Large Language Models
Conference on Language Modeling (COLM), Oct. 2024. -
Fujii, K., Nakamura, T., Loem, M., Iida, H., Ohi, M., Hattori, K., Hirai, S., Mizuki, S., Yokota, R., Okazaki, N.
Continual Pre-Training for Cross-Lingual LLM Adaptation: Enhancing Japanese Language Capabilities
Conference on Language Modeling (COLM), Oct. 2024. -
Otani, G., Tadokoro, R., Yamada, R., Asano, Y., Laina, I., Rupprecht, C., Inoue, N., Yokota, R., Kataoka, H., Aoki, Y.
Rethinking Image Super-Resolution from Training Data Perspectives
European Conference on Computer Vision (ECCV), Sep. 2024. -
Nakamura, R., Tadokoro, R., Yamada, R., Asano, Y., Laina, I., Rupprecht, C., Inoue, N., Yokota, R., Kataoka, H.
Scaling Backwards: Minimal Synthetic Pre-training?
European Conference on Computer Vision (ECCV), Sep. 2024. -
Yamada, R., Hara, K., Kataoka, H., Makihara, K., Inoue, N., Yokota, R., Satoh, Y.
Formula-Driven Visual-Geometric Pre-training
European Conference on Computer Vision (ECCV), Sep. 2024. -
Shen, Y., Daheim, N., Marconi, G. M., Nickl, P., Cong, B., Clement, B., Yokota, R., Gurevych, I., Cremers, D., Khan, M. E., Möllenhoff, T.
Variational Learning is Effective for Large Deep Networks
The 41st International Conference on Machine Learning (ICML), Jul. 2024.