TOP
Research
Research Teams
Supercomputing Performance Research Team
Supercomputing Performance Research Team
Japanese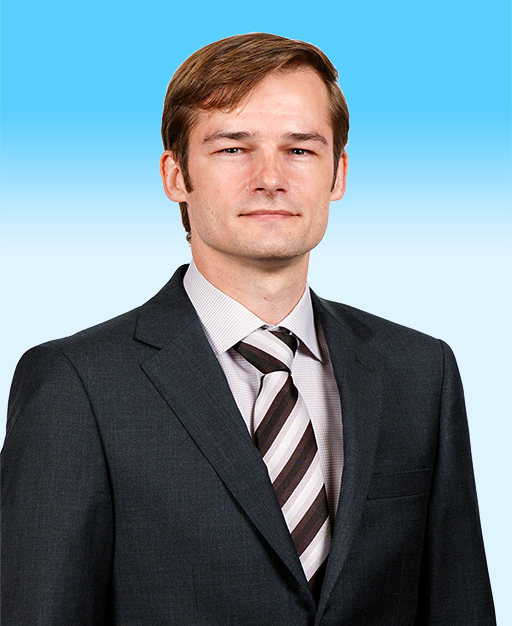
Team Principal Jens Domke
jens.domke[at]riken.jp (Lab location: Kobe)
- Please change [at] to @
- 2022
- Team Leader, Supercomputing Performance Research Team, R-CCS, RIKEN (-present)
- 2021
- Research Scientist, High Performance Big Data Research Team, R-CCS, RIKEN
- 2019
- Postdoctoral Researcher, High Performance Big Data Research Team, R-CCS, RIKEN
- 2019
- Tokyo Tech Research Fellow, Tokyo Institute of Technology
- 2017
- Postdoctoral Researcher, Matsuoka & Endo Laboratory, Global Scientific Information and Computing Center, Tokyo Institute of Technology
- 2017
- Doctor rerum naturalium, Faculty of Computer Science, Technische Universität Dresden
Keyword
- Performance Modelling and Predictions
- Hardware/Software Co-Design for HPC
- Architecture and Application Evaluations
- Instrumentation and Monitoring Tools
- Auto-Tuning and Portability
Research summary
The complexity of modern supercomputers is steadily increasing. Previously, we were able to ride the wave of persistent transistor shrinking as observed by G. Moore, and hence could focus on finding technological solutions for the ever growing need for supercomputing performance. But nowadays, utilizing these machines effectively and efficiently is becoming ever more challenging.
To tackle these challenges, and to provide our HPC users with the best and fastest scientific instrument for their modelling and simulation of real-world phenomena, our team is applying, researching, and developing state-of-the-art methodologies to analyze hardware options. We are implementing novel performance monitoring and analysis tools and are conducting detailed performance studies of HPC architectures and software subsystems. Our team's mission is to bring performance to the masses. With the right tools, automatic performance tuning frameworks, and appropriate co-design, we are able to enhance the user experience for Fugaku and we are able to design the next Japanese flagship supercomputers to meet the needs of our domain experts and researchers without them requiring an advanced degree in computer science.
Main research results
Matrix engines or units, in different forms and affinities, are becoming a reality in modern processors; CPUs and otherwise. The current and dominant algorithmic approach to Deep Learning merits the commercial investments in these units, and deduced from the No. 1 benchmark in supercomputing, namely High Performance Linpack, one would expect an awakened enthusiasm by the HPC community, too.
Hence, our goal is to identify the practical added benefits for HPC and machine learning applications by having access to matrix engines. For this purpose, we perform an in-depth survey of software stacks, proxy applications and benchmarks, and historical batch job records. We provide a cost-benefit analysis of matrix engines, both asymptotically and in conjunction with state-of-the-art processors. While our empirical data will temper the enthusiasm, we also outline opportunities to "misuse" these dense matrix-multiplication engines if they come for free.
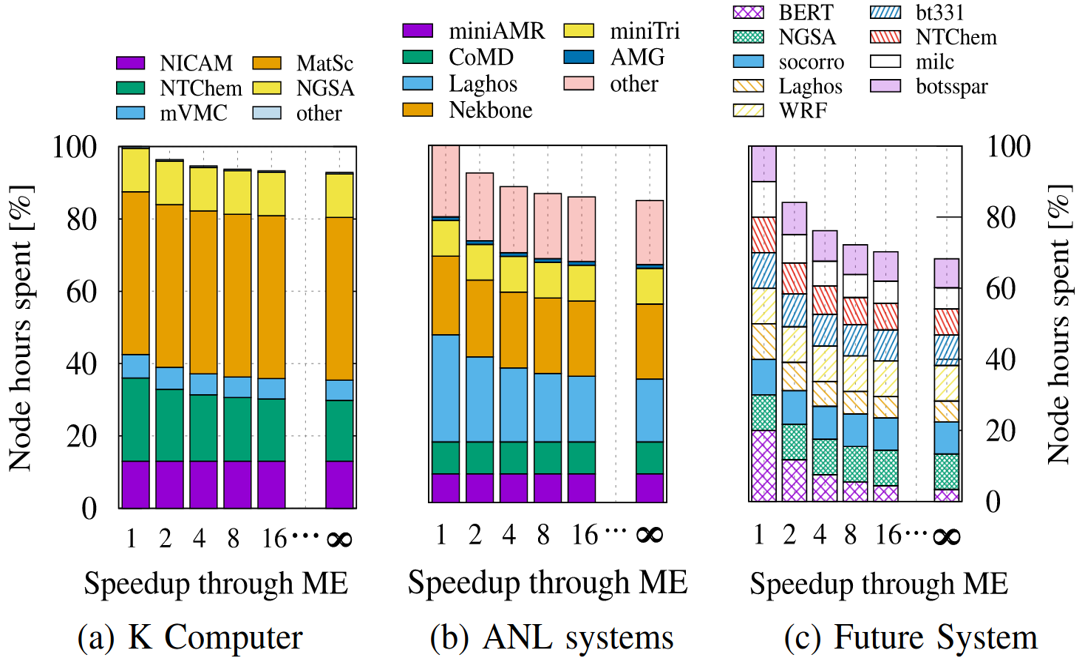
Representative papers
- T.N. Truong, F. Trahay, J. Domke, A. Drozd, E. Vatai, J. Liao, M. Wahib, B. Gerofi,
"Why Globally Re-shuffle? Revisiting Data Shuffling in Large Scale Deep Learning,"
in Proceedings of the 36th IEEE International Parallel & Distributed Processing Symposium (IPDPS), (Lyon, France), IEEE Computer Society, May 2022. - J. Domke, E. Vatai, A. Drozd, P. Chen, Y. Oyama, L. Zhang, S. Salaria, D. Mukunoki, A. Podobas, M. Wahib, S. Matsuoka,
"Matrix Engines for High Performance Computing: A Paragon of Performance or Grasping at Straws?,"
in Proceedings of the 35th IEEE International Parallel & Distributed Processing Symposium (IPDPS), (Portland, Oregon, USA), IEEE Computer Society, May 2021. - M. Besta, J. Domke, M. Schneider, M. Konieczny, S.D. Girolamo, T. Schneider, A. Singla, T. Hoefler,
"High-Performance Routing with Multipathing and Path Diversity in Supercomputers and Data Centers,"
IEEE Transactions on Parallel and Distributed Systems, vol. 32, no. 4, pp. 943-959, 2021. - M. Wahib, H. Zhang, T.T. Nguyen, A. Drozd, J. Domke, L. Zhang, R. Takano, S. Matsuoka,
"Scaling Distributed Deep Learning Workloads beyond the Memory Capacity with KARMA,"
in Proceedings of the International Conference for High Performance Computing, Networking, Storage and Analysis, SC ’20, (Piscataway, NJ, USA), IEEE Press, Nov. 2020. - J. Domke, S. Matsuoka, I.R. Ivanov, Y. Tsushima, T. Yuki, A. Nomura, S. Miura, N. McDonald, D.L. Floyd, N. Dube,
"HyperX Topology: First at-scale Implementation and Comparison to the Fat-Tree,"
in Proceedings of the International Conference for High Performance Computing, Networking, Storage and Analysis, SC ’19, (Piscataway, NJ, USA), IEEE Press, Nov. 2019. - J. Domke, K. Matsumura, M. Wahib, H. Zhang, K. Yashima, T. Tsuchikawa, Y. Tsuji, A. Podobas, S. Matsuoka,
"Double-precision FPUs in High-Performance Computing: an Embarrassment of Riches?,"
in Proceedings of the 33th IEEE International Parallel & Distributed Processing Symposium (IPDPS), (Rio de Janeiro, Brazil), IEEE Computer Society, May 2019. - S. Smith, C. Cromey, D.K. Lowenthal, J. Domke, N. Jain, J.J. Thiagarajan, A. Bhatele,
"Mitigating Inter-Job Interference Using Adaptive Flow-Aware Routing,"
in Proceedings of the International Conference for High Performance Computing, Networking, Storage and Analysis, SC ’18, (Piscataway, NJ, USA), IEEE Press, Nov. 2018. - J. Domke and T. Hoefler,
"Scheduling-Aware Routing for Supercomputers,"
in Proceedings of the International Conference for High Performance Computing, Networking, Storage and Analysis, SC ’16, (Piscataway, NJ, USA), pp. 13:1-13:12, IEEE Press, 2016. - J. Domke, T. Hoefler, and S. Matsuoka,
"Routing on the Dependency Graph: A New Approach to Deadlock-Free High-Performance Routing,"
in Proceedings of the 25th ACM International Symposium on High-Performance Parallel and Distributed Computing, HPDC ’16, (New York, NY, USA), pp. 3-14, ACM, 2016. - J. Domke, T. Hoefler, and S. Matsuoka,
"Fail-in-place Network Design: Interaction Between Topology, Routing Algorithm and Failures,"
in Proceedings of the International Conference for High Performance Computing, Networking, Storage and Analysis, SC ’14, (Piscataway, NJ, USA), pp. 597-608, IEEE Press, 2014.